How AI reinforces gender bias—and what we can do about it
Interview with Zinnya del Villar on AI gender bias and creating inclusive technology
Date:
AI is transforming our world—but when it reflects existing biases, it can reinforce discrimination against women and girls. From hiring decisions to healthcare diagnoses, AI systems can amplify gender inequalities when trained on biased data. So how can we ensure AI is ethical and inclusive? Zinnya del Villar, a leading expert in responsible AI, shares insights on the challenges and solutions in a recent conversation with UN Women.
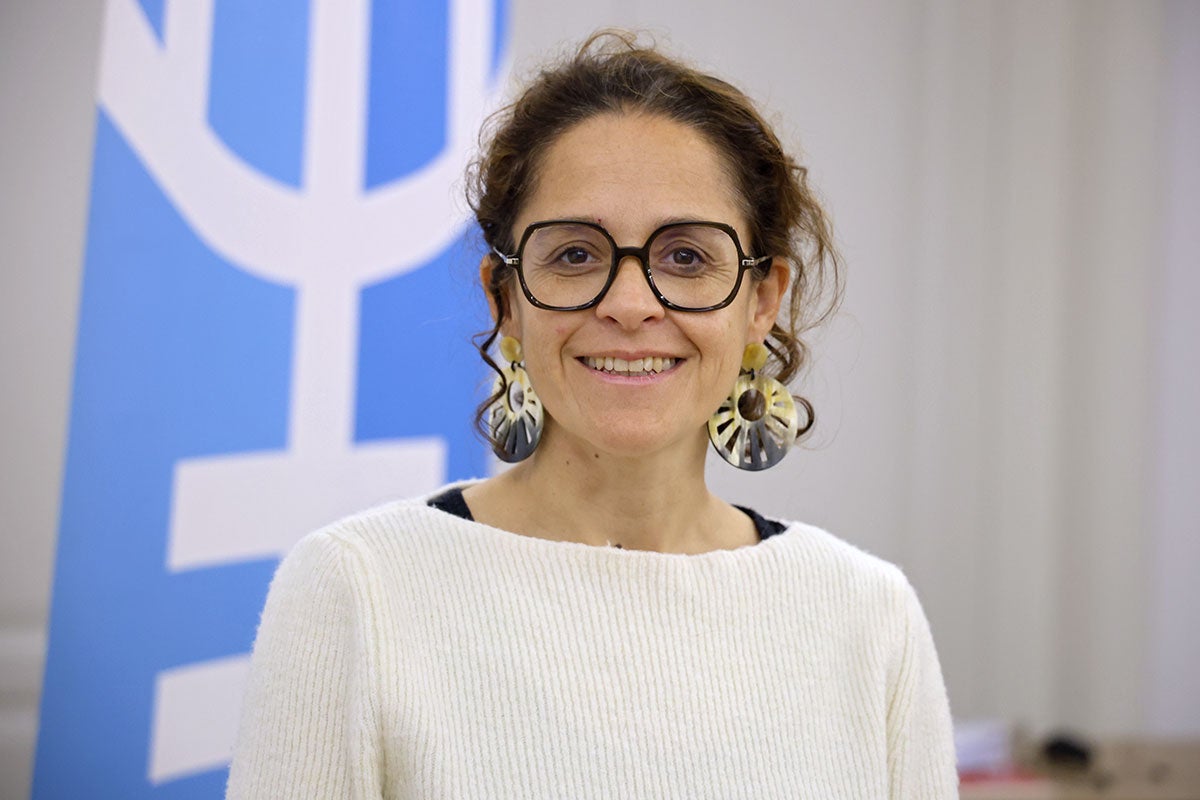
What is AI gender bias and why does it matter?
“AI systems, learning from data filled with stereotypes, often reflect and reinforce gender biases,” says Zinnya del Villar. “These biases can limit opportunities and diversity, especially in areas like decision-making, hiring, loan approvals, and legal judgments.”
At its core, Artificial Intelligence – or AI – is about data. It is a set of technologies that enable computers to do complex tasks faster than humans. AI systems, such as machine learning models, learn to perform these tasks from the data they are trained on. When these models rely on biased algorithms, they can reinforce existing inequalities and fuel gender discrimination in AI.
Imagine, training a machine to make hiring decisions by showing it examples from the past. If most of those examples carry conscious or unconscious bias – for example, showing men as scientists and women as nurses – the AI may interpret that men and women are better suited for certain roles and make biased decisions when filtering applications.
This is called AI gender bias— when the AI treats people differently on the basis of their gender, because that’s what it learned from the biased data it was trained on.
What are the impacts of gender bias in AI applications?
Gender bias in AI has deep consequences in real life.
“In critical areas like healthcare, AI may focus more on male symptoms, leading to misdiagnoses or inadequate treatment for women,” shares del Villar. “Voice assistants defaulting to female voices reinforce stereotypes that women are suited for service roles, and language models like GPT and BERT often associate jobs like "nurse" with women and "scientist" with men.”
Del Villar turns to some examples of AI gender bias that have been well documented: In 2018, Amazon discontinued an AI recruitment tool that favoured male resumes. Image recognition systems from companies have struggled to accurately identify women, particularly women of colour, leading to mistaken identifications, which can have serious consequences in law enforcement and public safety.
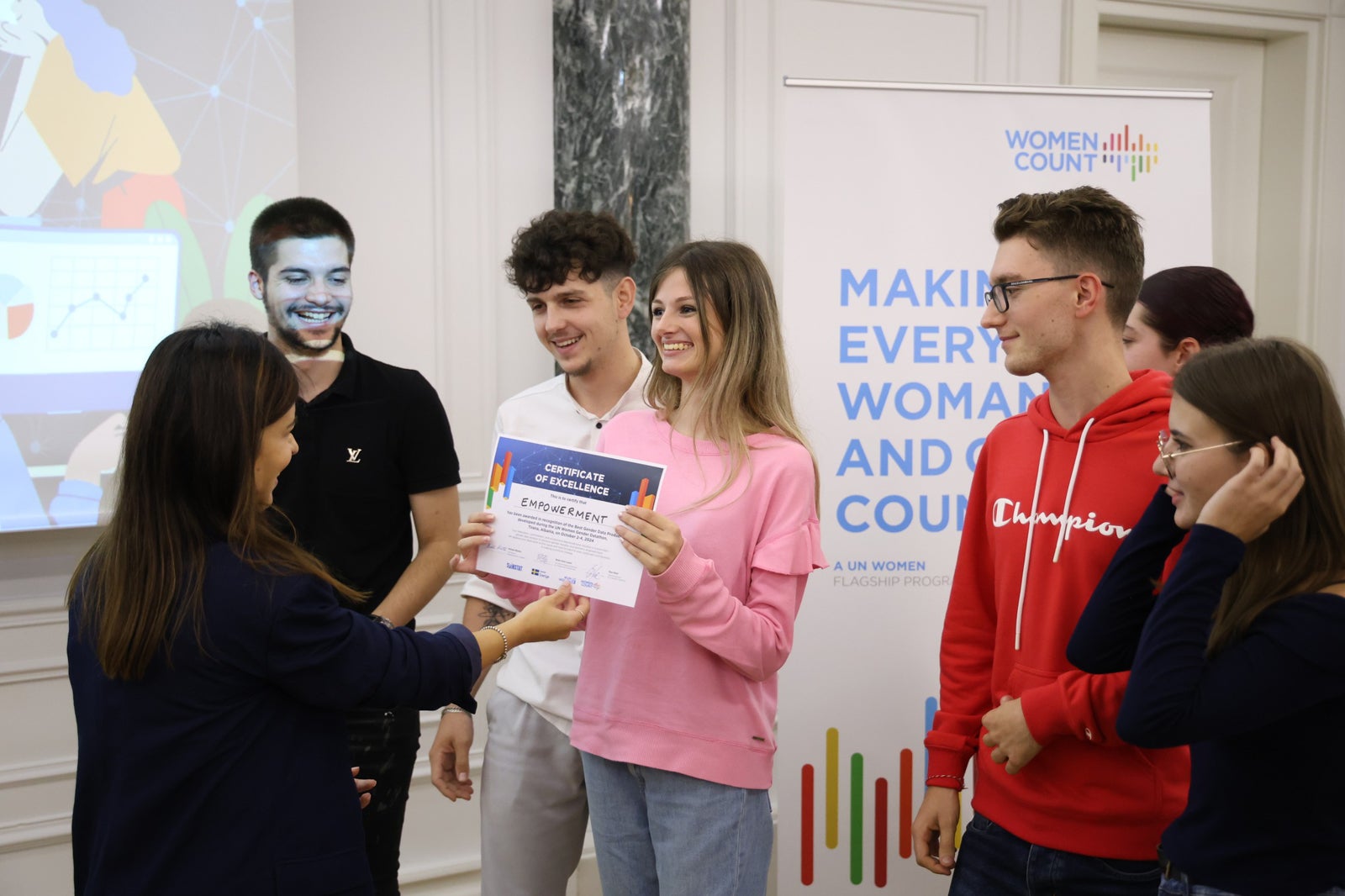
How can we reduce gender bias in AI systems?
Artificial Intelligence mirrors the biases that are present in our society on the basis of gender, age, race, and many other factors.
“To reduce gender bias in AI, it’s crucial that the data used to train AI systems is diverse and represents all genders, races, and communities,” stresses del Villar. “This means actively selecting data that reflects different social backgrounds, cultures and roles, while removing historical biases, such as those that associate specific jobs or traits with one gender.”
“Additionally, AI systems should be created by diverse development teams made up of people from different genders, races, and cultural backgrounds. This helps bring different perspectives into the process and reduces blind spots that can lead to biased AI systems.”
Public awareness and education are essential parts of this strategy, adds del Villar. Helping people understand how AI works and the potential for bias can empower them to recognize and prevent biased systems, and keep human oversight on decision-making processes.
How can AI help identify gender bias and drive better decisions?
Although AI-generated data carries risks of gender bias, it also holds significant potential for identifying and addressing gender inequalities across sectors. For example, AI has helped analyze large amounts of data to find gender pay gaps in the workforce, with tools like Glassdoor showing differences in salaries based on gender.
In finance, AI is helping overcome long-standing gender biases in credit scoring, as seen with companies like Zest AI, which use machine learning to make fairer credit assessments. AI is also improving access to microfinance services for women entrepreneurs to access loans and financial services, particularly in underserved areas.
AI has helped reveal the disparity in enrollment rates between men and women on platforms such as Coursera and edX, and uncovered biases in textbooks, helping educators revise learning materials to be more inclusive.
“AI is tracking gender representation in leadership roles and encouraging the use of gender quotas to address inequalities ,” shares Villar. “It can also assist in analyzing and drafting gender-sensitive laws by identifying patterns of gender discrimination and proposing reforms. In the future, AI could help governments assess the potential gender impacts of proposed laws and help prevent gender discrimination and inequity.”
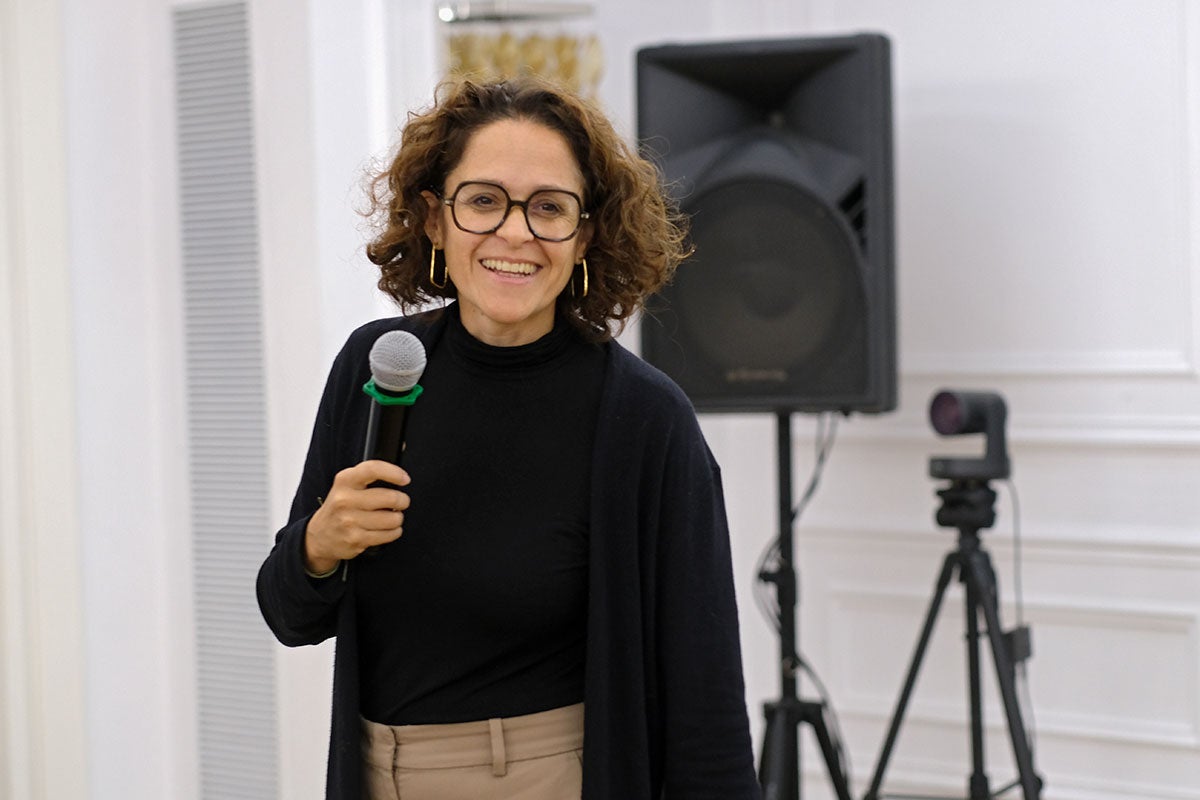
How can AI improve women’s safety and stop digital abuse?
While technology-facilitated violence against women and girls online and offline is a growing concern, there are many promising advancements in AI offering innovative solutions to address digital abuse and protect survivors.
For example, mobile apps like bSafe provides safety alerts to protect women, while Canada-based Botler.ai helps victims understand if sexual harassment incidents they experienced violate the U.S. criminal code or Canadian law. Chatbots like “Sophia” by Spring ACT and “rAInbow” by AI for Good provide anonymous support and connect survivors with legal services and other resources.
“AI-powered algorithms can also be used to make the digital space safe for everyone by detecting and removing harmful, discriminatory content and by stopping the spread of non-consensual intimate images,” adds Villar.
Five steps to more inclusive AI systems
Artificial Intelligence can be used to reduce or perpetuate the biases and inequalities in our societies. Here are five steps that Villar recommends to make AI inclusive – and better.
- Using diverse and representative data sets to train AI systems
- Improving the transparency of algorithms in AI systems
- Making sure AI development and research teams are diverse and inclusive to avoid blind spots
- Adopting strong ethical frameworks for AI systems
- Integrating gender-responsive policies in developing AI systems
About Zinnya del Villar
Zinnya del Villar is the Director of Data, Technology, and Innovation at Data-Pop Alliance. She advocates for women and girls in Science, technology, engineering, and mathematics (STEM) and ethical and inclusive use of data in Artificial Intelligence (AI) systems. She is collaborating with UN Women on researching the gendered impacts of the war in Ukraine and on enhancing gender data literacy in the region, including through the Making Every Woman and Girl Count programme. She is listed among the 100 Brilliant Women in AI Ethics - 2024.